Success stories
VOO – BI & Big Data Transformation & Migration to the Cloud
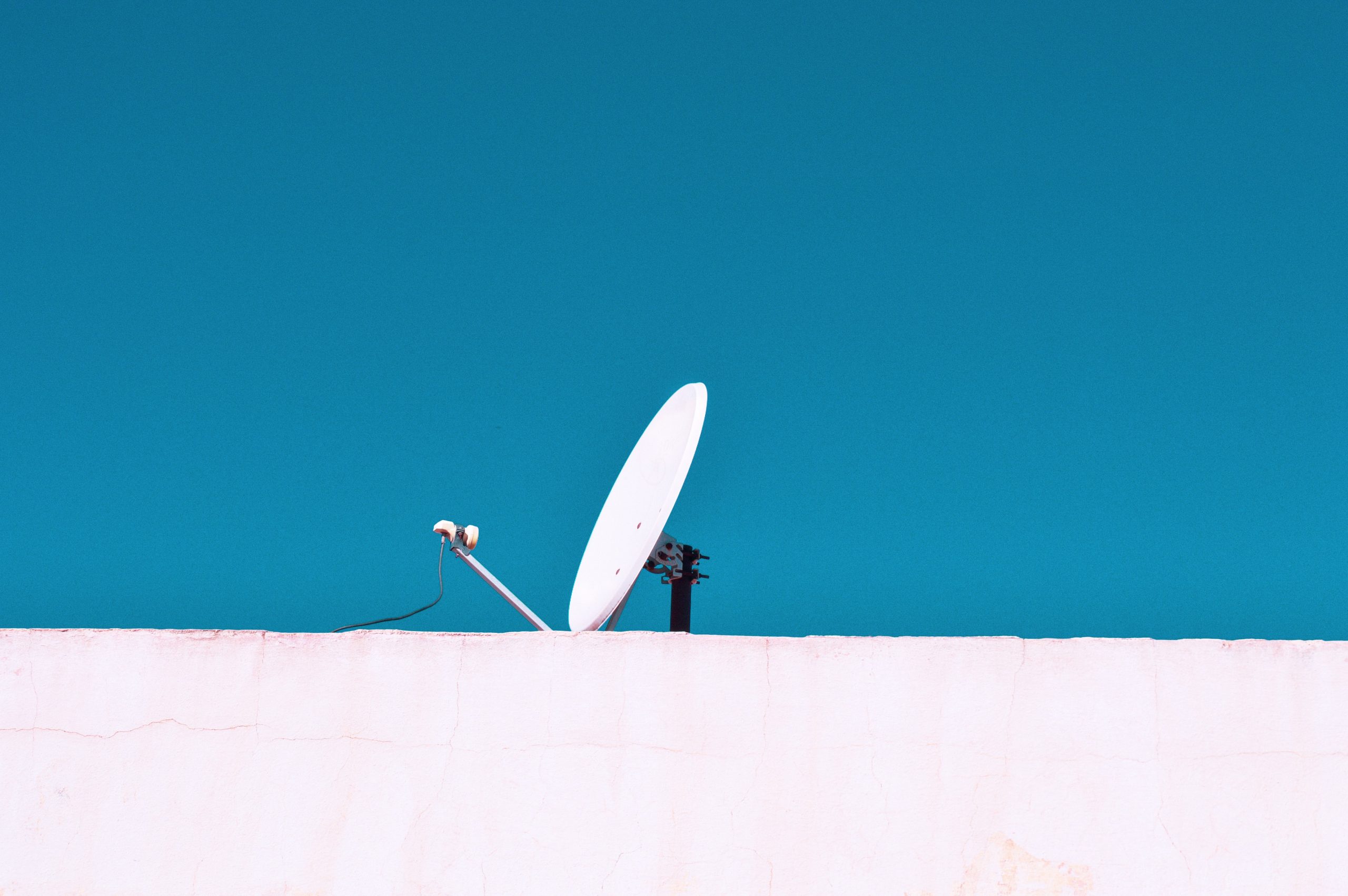
June 3, 2020
VOO – one of Belgium’s largest telecom operators on the market – completed Memento. What is it? It is Voo’s Business Intelligence and Big Data transformation program with a key migration to the Cloud. Find out more about how we assisted the operator during the different phases of the project.
Who is VOO?
Voo is the name of a Belgian Telecom operator. They’re mainly active in the regions of Wallonia and Brussels. Providing their customers with cable, telephone and internet services, Voo is customer oriented and technology savvy.
You and your whole family can enjoy super fast broadband Internet, a rich mine of TV content and a generous mobile and landline service thanks to the innovative products and services of VOO. They’re a Belgian telecom operator that the experts of Lucy helped with their Digital Transformation.
What was the challenge that VOO approached Lucy with?
In the context of global transformation, we implemented a complete migration of VOO’s Business Intelligence, Big Data, and AI landscape to the Cloud. This migration was critical to meet strategic and urgent business needs such as:
- Dramatically increase customer insights to speed up acquisition and improve loyalty and retention
- Support the digital transformation by offering a unified vision of the customer and his behavior
- Meet the new compliancy challenges (GDPR)
- Radically reduce overall data environments TCO (4 different BI environments + 3 Hadoop clusters before the transformation)
- Introduce company-wide data governance and address shadow BI (25+ FTE’s on the business side crunching and processing data)
Our solution: a Cloud-based, Enterprise-wide data platform powered by AWS
Experts at Lucy conducted a rapid study, scanning all aspects of the transformation and addressing both the organizational challenge (Roles and responsibilities, teams and skills, processes, governance) and the technical challenge (holistic architectural scenarios, ranging from hybrid cloud to full cloud solutions in PaaS mode).
Based on the outcome of the study, we deployed a Cloud-based, Enterprise-wide data platform. It combines traditional BI processes with advanced analytical capabilities. We redefined the data organization and related processes and introduced data governance at corporate level.
The TCO dropped to less than 30% of what it used to be. Agility and capabilities dramatically improved.
Architecture based upon key data services of AWS
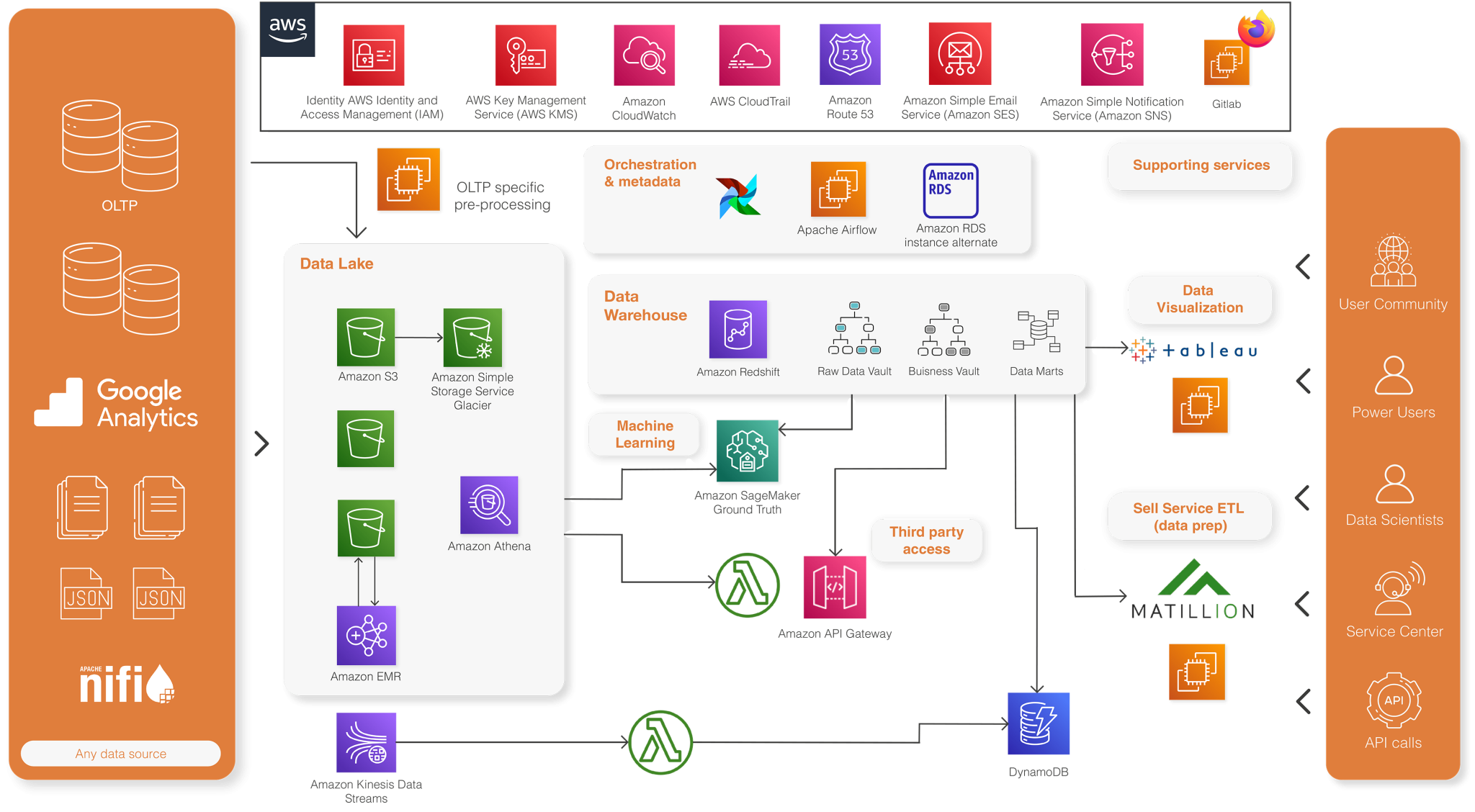
Data Lake
Amazon S3 is used for central inbound layer and achieve long term persistence.
Some data files are pre-processed on Amazon EMR. EMR clusters are created on-the-fly a couple of times per day. The clusters only process new data that arrive in S3. Once the data is processed and persisted in an analytical optimized Apache Parquet format, the cluster is destroyed. Encryption and lifecycle management are enabled on most S3 buckets to meet security and cost-efficiency requirements. The data is currently stored in the Data Lake. Amazon Athena is used to create and maintain a data catalog and explore raw data in the Data Lake.
Data Warehouse
The Data Warehouse is running on Amazon Redshift, using the new RA3 nodes and follows the Data Vault 2.0 methodology. Data Vault objects are very standardized and have strict modeling rules, which allows a high level of standardization and automation. The data model is generated based on metadata stored in an Amazon RDS Aurora database.
The automation engine itself is built on AWS Step Functions and AWS lambda.
DynamoDB
Amazon DynamoDB is being used for specific use cases where web-applications need sub-second response times. Using the DynamoDB’s variable read/write capacity allows provisioning the more expensive high performance read capacity during business hours only, where low latency and fast response time are required. Such mechanisms, which rely on the AWS services’ elasticity, are used to optimize the AWS monthly bill.
Machine Learning
A series of predictive models have been implemented, ranging from a classical churn prediction model to more advanced use cases. Amazon SageMaker was used to build, train, and deploy the models at scale, leveraging the data available in the Data Lake (Amazon S3) and the Data Warehouse (Amazon Redshift).
And much more!
The Data Platform we have built offers dozens of other capabilities. The huge set of services available on the AWS environment allows addressing new use cases every day, in a fast and efficient way.